About Us
Mantis was founded with the aim of doing interesting and impactful work. As a company we hold a number of beliefs which define our culture and our values.
We believe
Experiments should be easily reproducible. This encourages more trust in the results, enables better collaboration within a team, and reduces duplication of work.
Open source software and practices have made, among other things, the AI revolution possible. They accelerate progress, and encourage collaboration between people and organisations. We use open source by default, and contribute to the open source community where possible.
Data reflect human biases. AI and as an extension Natural Language Processing applications mimic those biases and can cause unintended harm. Detecting and reducing ethical risks is the responsibility of all data scientists.
It’s our responsibility to combat climate change to support a more sustainable way of life. Training large deep learning models for Natural Language Processing tasks can have enormous carbon footprints so we avoid using such models where possible, and use computing resources responsibly and efficiently.
AI and NLP is not a neutral technology. Applying Natural Language Processing tools for military, political or marketing purposes has an impact on our democracy and free will. We think carefully about the impact our work might have, and are selective about the industries and clients we work with.
Leadership Team
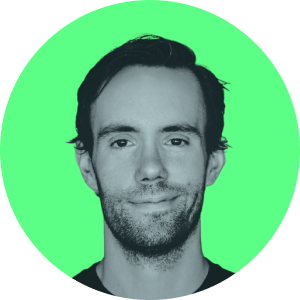
Dr. Matthew Upson
After completing his PhD in environmental science, Dr. Matthew Upson shifted his focus to Natural Language Processing (NLP) and has been working on related problems since 2015. His career has taken him through various roles as a data scientist in UK Government departments, startups, and NGOs. His work on reproducibility in Official Statistics was recognized and included in a new Government strategy in 2022. Beyond his professional roles, Matt is a founding member of the Royal Statistical Society's Data Science Section and a fellow of the Software Sustainability Institute. He is also the author of an upcoming book on 'Open Source MLOPs', set to be published in late 2023.
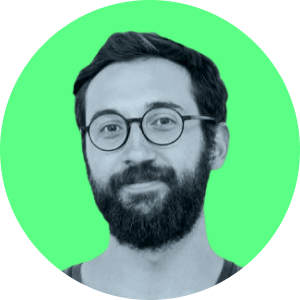
Nick Sorros
Nick Sorros, a graduate of Imperial College London with a MSc in Computer Science, has been working as a data scientist since 2013. His career has spanned numerous London-based startups, eventually leading him to a leadership role at the Wellcome Trust. Nick shifted his focus from Computer Vision to NLP when he transitioned to industry and has since worked with datasets of varying sizes and complexities. Nick has a particular interest in reproducible, reusable, and open-source code. Outside of work, he volunteers for DataKind UK and often assists with the organization of PyData London.
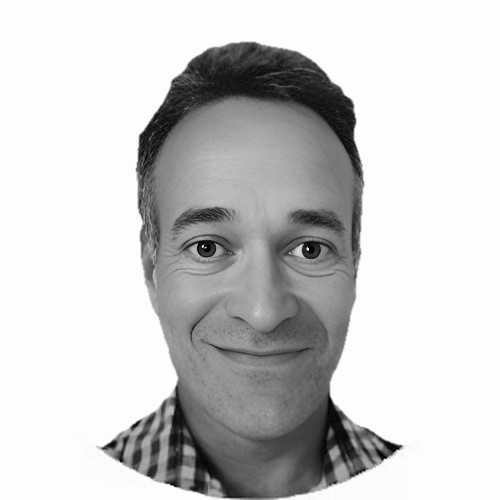
Doug Ayres
Doug Ayres is a strategic business leader and current CEO of EBM, a chatbot technology and services business spun out of Filament Consulting Group. At EBM, Doug oversees the delivery of comprehensive chatbot solutions, incorporating advanced technology and professional services. Doug co-founded Filament Consulting Group in 2016, a software consultancy specializing in machine learning technologies. His earlier ventures include FUSE, a digital agency he co-founded in 2004, which was later sold to IPG in 2011. Doug provides strategic advice to the leadership team.